Clinical Cognition in the Context of Artificial Intelligence
- In ICU
- Mon, 5 Aug 2024
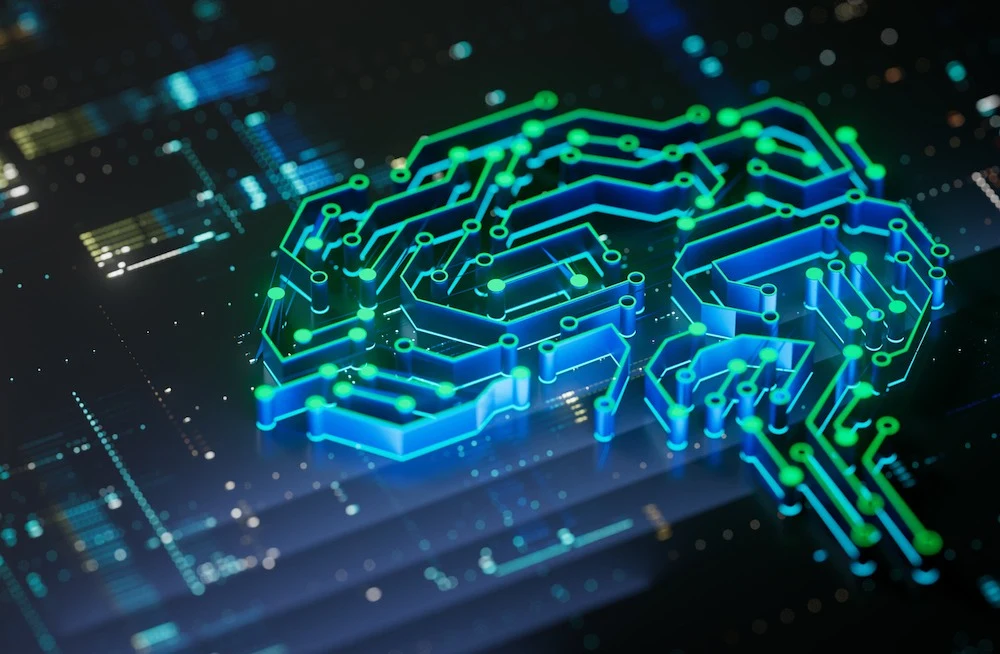
Medical decision systems powered by artificial intelligence (AI) are widely used in healthcare. The rapid development and commercialisation of these systems outpace the understanding of their clinical value, creating an "AI chasm." This gap is due to various technical and logistical challenges and issues in clinical implementation.
The relationship between humans and machines can lead to over-reliance, under-reliance, and overwhelm. Current research on the clinician-AI relationship is often based on self-reported and superficial analysis. To address these challenges and bridge the AI chasm, cognitive science and human factors insights can provide a deeper understanding of human decision-making in clinical contexts.
Clinicians diagnose by detecting cues in the clinical environment, which are sensory signals that direct their attention and activate abstract knowledge representations called inner cognitive cues. These cues, often imperceptible to novices, are crucial for diagnosis. As clinicians gain experience, they develop the skill of cue utilisation, which involves focusing on the most clinically relevant information. This expertise allows them to quickly grasp the essential features of a clinical scene with high accuracy, guiding further analysis. Similar processes include clinical gestalt, where patterns of signs and symptoms intuitively indicate a medical issue. Such decision-making methods, which utilise the surrounding context to simplify tasks, are as effective as more complex methods—a concept known as ecological rationality.
Rationality is an approach to decision-making based on formal reasoning, often involving abstract mathematical rules like logic and probability. However, humans face sensory, cognitive, and time limitations, making it challenging to optimise every decision perfectly. Instead, people often "satisfice," meaning they make decisions that are good enough based on the available information. By using environmental cues, clinicians can narrow down the range of potential decisions, allowing for efficient decision-making based on context rather than complete knowledge of all possible options and outcomes.
Unlike humans, new deep-learning AI systems can handle vast amounts of information for decision-making without the same constraints. However, AI lacks the ability to disregard irrelevant cues, which can simplify decision-making for humans. AI systems also cannot question the validity of their data, unlike clinicians who practice epistemic humility by critically evaluating their knowledge. This fundamental difference in decision-making capabilities between AI and clinicians extends across healthcare and AI development.
The distinction between human and AI decision-making, particularly in terms of rationality and the ability to handle contextual cues, highlights the complexity of integrating AI into clinical practice. This complexity is often underestimated, suggesting that the integration process is more challenging than currently recognised.
The ecologically bounded model of cognition suggests that rational and optimal decision-making is based on environmentally valid inferences. In this context, bounded rationality refers to a clinician's ability to be accurate using limited information. The concept of debounding describes the opposite process, where decisions are made using all available information, even if it's not optimal or comprehensible for the clinician. Debounding from ecological features can lead AI models to rely on irrational features, a phenomenon known as shortcut learning. This occurs when models use irrelevant cues to make diagnoses.
AI, however, is not perfectly traditionally rational due to the limitations of its training data. Models produce decisions based on cues from the training data, which may not be clinically valid. These decisions become "dataset-bounded," relying on any feature correlated with the labels rather than clinically useful information. As AI models improve in accuracy, they may diverge further from human decision-making processes. This mismatch raises concerns about black box problems and model explainability, where understanding the reasoning behind AI decisions becomes challenging.
The interaction between clinicians and AI is complex due to the differences in decision-making processes and capabilities. AI systems often present decisions using different logic from humans, making it difficult for clinicians to understand the underlying information used by AI. This lack of understanding introduces vulnerabilities, such as difficulty anticipating AI errors or biases. Clinicians face the challenge of balancing over-reliance on AI outputs and under-reliance, where they might avoid AI altogether.
A deeper understanding of cognition in the context of AI is needed, going beyond observable behaviours to study underlying cognitive processes. This approach, which includes behavioural, cognitive, and cognitive models of analysis, can help understand how clinical decision-making adapts in response to AI.
The concept of clinicians and AI as a synergistic team is misleading because human teams rely on shared understanding and cognitive mechanisms, while human AI teams do not. Clinicians are ecologically bounded, making decisions based on their knowledge and environment, whereas AI is dataset-bounded, learning from correlations in training data without contextual limitations. For the safe development and implementation of medical AI, a comprehensive understanding of clinical cognition in the context of AI use is essential.
As deep-learning technologies become more autonomous and complex, yet often misleadingly human-like in presentation, it's crucial to shift the approach to clinician cognition in AI use. This shift involves recognising the role of cognitive decision-making in model development and model use, highlighting the fundamental differences between human and AI decision-making, and expanding research to include cognitive, environmental, and neurophysiological aspects of decision-making. This comprehensive approach will better address the internal complexities and ensure safer integration of AI in medical settings.
Source: The Lancet Digital Health
Image Credit: iStock
References:
Tikhomirov L, Semmler C, McCradden M et al. (2024) Medical artificial intelligence for clinicians: the lost cognitive perspective. Lancet Digit Health. 6(8):e589-e594.