Impact of Machine Learning on Predicting Clinical Deterioration
- In ICU
- Tue, 23 Jul 2024
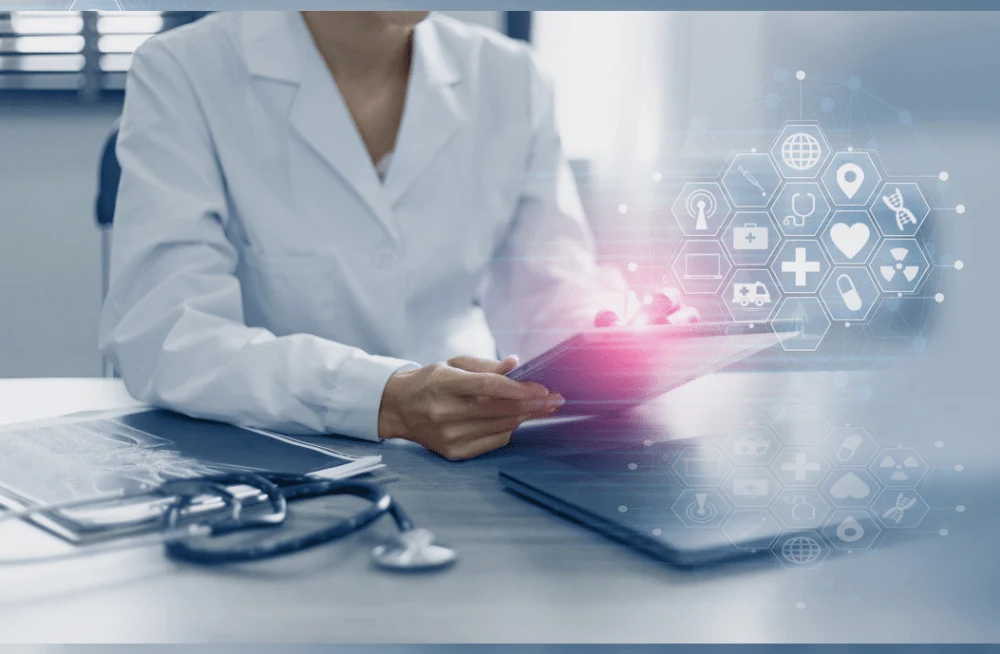
Machine learning algorithms are transforming healthcare by enhancing the prediction of clinical deterioration. Despite their potential, real-world efficacy data remains limited. A recent study hypothesised that real-time machine learning-generated alerts, sent directly to front-line providers, could significantly reduce patient escalations. A recent article published in Critical Care Medicineexplores the implications for the future of healthcare.
Study Design and Setting
The study was conducted at an academic tertiary care medical centre, involving adult patients admitted to four medical-surgical units. Based on initial unit admission, the single-centre, prospective, pragmatic nonrandomised clustered clinical trial assigned patients to either intervention or control groups. The primary objective was to assess if real-time alerts indicating potential deterioration could reduce the escalations rate.
Interventions and Methodology
In the intervention units, real-time alerts stratified by the likelihood of deterioration were sent to either the primary care team or directly to the rapid response team (RRT). Clinical care decisions remained at the discretion of the providers. In the control units, while alerts were generated, they were not sent, and standard RRT activation criteria were followed. The primary measurement was the escalation rate per 1000 patient bed days, with secondary outcomes including the frequency of orders for fluids, medications, diagnostic tests, and combined in-hospital and 30-day mortality.
Key Findings and Results
Data from 2740 patients (1488 intervention, 1252 control) were analysed. The intervention group had a higher rate of escalations (12.3 vs. 11.3 per 1000 patient bed days) with an incidence rate ratio of 1.43. The intervention group saw increased cardiovascular medication orders (16.1% vs. 11.3%) and a significantly lower combined in-hospital and 30-day mortality rate (7% vs. 9.3%). These results highlight the effectiveness of real-time machine learning alerts in prompting earlier and more appropriate interventions, ultimately improving patient outcomes.
This study underscores the potential of machine learning algorithms to revolutionise patient care by more accurately predicting clinical deterioration than traditional methods. Implementing real-time alerts led to timely and enhanced medical responses, reducing mortality rates among hospitalised patients. As AI technology continues to evolve and integrate into healthcare systems, it promises to further improve patient outcomes, making it an indispensable tool in modern medicine.
Source Credit: Critical Care Medicine
Imagr Credit: iStock